Closed-loop optimization of fast-charging protocols for batteries with machine learning
This post is a review of “Closed-loop optimization of fast-charging protocols for batteries with machine learning” (2019) by Attia, Grover, Jin, Severson, Markov, Liao, Chen, Cheong, Perkins, Yang, Herring, Aykol, Harris, Braatz, Ermon, and Chueh.
Closed-loop optimisation (CLO) helps to find an optimal fast-charging protocol quickly, assuming only a limited number of experiments can run in parallel. The two pillars of CLO are the early outcome predictor and Bayesian optimization:
Testing a single battery to failure under our fast-charging conditions requires approximately 40 days, meaning that when 48 experiments are performed in parallel, assessing all 224 charging protocols with triplicate measurements takes approximately 560 days.
The authors assume a constraint that only 48 experiments can run in parallel. This is why they need the Bayesian optimisation component in CLO to make the search faster. This seems like an artificial limitation to me, because in practice, a Li-ion cell or a car manufacturer can dedicate more than 48 cell cycling chambers to find the optimal fast-charging protocol which will later be used for millions of cells.
However, Li-ion manufacturers may apply the basic framework from this paper in their process and materials research, because they can run only a few cell material experiments in parallel.
The authors constrain the fast-charging protocol design space in the following way: They only decide distinct charging rates for 0–20%, 20–40%, 40–60% SoC ranges (called CC1, CC2, CC3 respectively). The CC4 rate in 60–80% SoC range is determined by CC1, CC2, CC3, and the constraint that the protocol should take exactly 10 minutes for charging from 0% to 80%. The constant-current rate for SoC > 80% is fixed to 1C, followed by a constant-voltage phase after 3.6V reached.
Key proposition: optimal fast-charging protocols are flat rather than decreasing by the charging rate
Most fast-charging protocols proposed in the battery literature suggest that current steps decreasing monotonically as a function of SOC are optimal to avoid Lithium plating on graphite, a well-accepted degradation mode during fast charging. In contrast, the protocols identified as optimal by CLO are generally similar to single-step constant-current charging (that is, CC1 ≈ CC2 ≈ CC3 ≈ CC4).
We hypothesize that solid-electrolyte interphase (SEI) growth, exacerbated by high temperatures, is the dominant degradation mode during these extreme operating conditions:
- While lithium plating is generally expected at high charging rates, the high environmental temperature (30°C) is a temperature regime in which lithium plating is unfavorable. The rate of heat generation during fast charging surpasses the convective cooling in the chamber, so the cell temperature increase during cycling is significant [see figure below the quote]. In general, side reaction rates increase exponentially with temperature from the Arrhenius relation.
- Resistive (Ohmic) heat generation is governed by I^2*R. We determined that the resistance is both Ohmic (linear overpotential vs. current relationship) and largely independent of SoC, particularly for minimally-cycled cells. For a constant R, I^2 is minimized via constant-current charging.
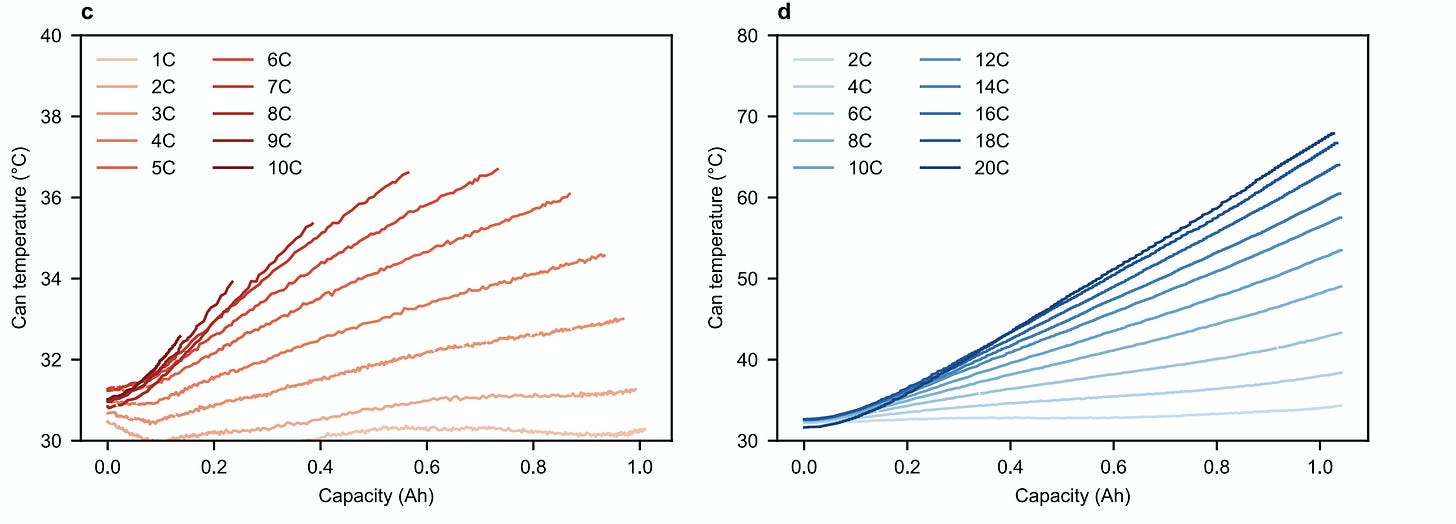
I want to note that the authors predict a cell's life from 100 fast charging cycles done in just 4 days. The discharge step (at 4C rate, i. e. 15 minutes to full discharge) follows the fast charging step with just 5 seconds of rest in between. Then the next charging loop starts after just another 5 seconds of rest! This non-stop fast charging and discharging puts the cell at the equilibrium temperature of probably at least 40 °C (the authors didn't measure the temperature during their main experiment; the above charts are from a separate lab test).
In such an experiment, the charging protocol that minimises the cell's equilibrium temperature may perform the best because accelerated parasitic reactions (such as the growth of the SEI layer on anode) due to the temperature of about 40 °C might contribute more to cell's degradation than Lithium plating.
But I wouldn't say this result refutes the "conventional battery wisdom" that Lithium plating is the leading degradation factor to look at in the design of real-life fast-charging protocols. The temperature increase from charging at, say, 6C (on the chart above; 30→36°C) doesn't look dramatic to me, assuming that the cell will have time to cool down when it is discharged slowly with intermittent rest (for example, during city driving) for many hours after the fast charging. Let alone there will likely be an active cooling system in the battery, trying to keep cells' temperatures at about 30 °C (?). So the high C-rates during fast-charging shouldn't noticeably affect the long-term average temperature of the cells.
Early estimator overestimates the cycle life of the cells
We attribute the source of this systematic bias to additional calendar ageing, which lowers the initial discharge capacities and thus leads to larger predictions. A similar effect was presented in Supplementary Figures 6 and 7 and Supplementary Note 2 of Severson et al. [2]
This is a strange statement because actually Severson et al. [2] observed the opposite effect: “...the calendar life effect reduces the predicted lifetime in the full model.” (Supplementary Note 2.) And here is their predictions plotted against the reality:
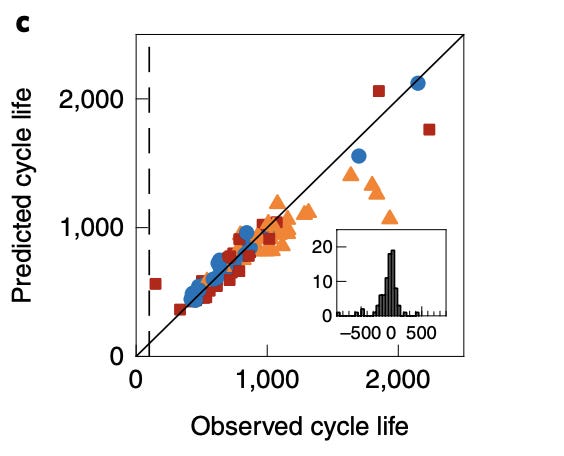
Orange triangles are visibly below the diagonal, but note how axes are swapped, compared to the chart from the CLO paper.
Cell data mining, analysis, and modelling is greatly complicated by factors that are not essential to the basic Li-ion cell electrochemistry
An important conclusion that I make for myself from this paper is that the data from cell experiments is often “tainted” by something accidental:
The main proposition of this paper, that optimal fast-charging protocols are flat rather than decreasing by the charging rate, might be due to the fact that the cells didn’t have time to cool down between the charge-discharge cycles during the experiment.
The model for early estimation of a cell’s life is biased probably by the shelf life of the cells on which this model was trained.
Cylindrical cell's discharge capacity can increase over the first few cycles not due to some fundamental electrochemical effects, but probably because of the geometry of cylindrical cells (the anode and cathode stripes have different lengths).
References
[1] "Closed-loop optimization of fast-charging protocols for batteries with machine learning" (2019)
[2] "Data-driven prediction of battery cycle life before capacity degradation" (2019)